The Ethics of Using Big Data and Analytics in Healthcare
- Posted on January 25, 2023
- By Vishakha Yadav
- Read 5 minutes
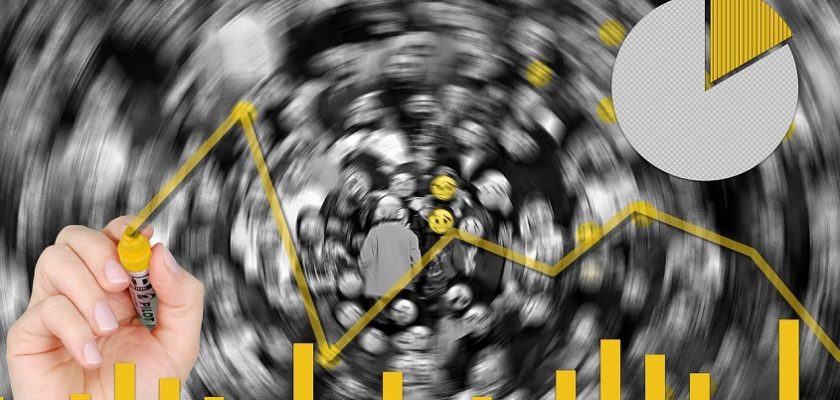
The use of big data and analytics in healthcare has the potential to greatly improve patient outcomes, increase efficiency, and drive advancements in research and development. However, there are also significant ethical concerns surrounding the use of this technology in healthcare, including issues related to privacy and security, bias and discrimination, and lack of transparency and accountability. Current regulations, such as HIPAA, aim to protect patient data, but there are still gaps in these protections. It is crucial for healthcare professionals to consider the ethical implications of using big data and analytics in their practice and to adhere to best practices, such as data governance, a patient-centric approach, and transparency. It’s also important to be aware of international considerations in this topic as well. Overall, while the use of big data and analytics in healthcare has the potential to greatly benefit patients, it is crucial that it is done in an ethical and responsible manner.
The benefits of using big data and analytics in healthcare
- Improved patient outcomes by identifying patterns, trends and insights that can help doctors and healthcare professionals make better-informed treatment decisions.
- Increased efficiency in healthcare operations by identifying bottlenecks and inefficiencies, and providing actionable insights to improve operations.
- Cost savings by reducing medical errors and unnecessary treatments, and identifying areas for cost savings.
- Advancements in research and development by providing large, diverse data sets that can be used to identify new drugs, therapies, and treatments.
- Personalized medicine by identifying patient subgroups that respond differently to treatment, and tailoring therapy accordingly.
- Predictive analytics by identifying patients at risk for certain conditions, allowing for early intervention and prevention.
- Improved population health management by identifying population-level health trends, and developing strategies to improve overall health outcomes.
- Streamlining administrative tasks by automating data entry, tracking patients and claims, and reducing the need for manual data entry.
The potential drawbacks and ethical concerns
- Privacy and security concerns by the risk of breaches and unauthorized access to sensitive patient information.
- Bias and discrimination by the potential for algorithms to perpetuate and amplify existing biases, especially if the data used to train them is biased.
- Lack of transparency and accountability by the black box nature of some AI/ML models, making it difficult to understand how decisions are being made.
- Fairness concerns by the potential to reinforce existing disparities in health outcomes among different population groups.
- Lack of transparency and accountability by the black box nature of some AI/ML models, making it difficult to understand how decisions are being made.
- Overreliance on data by the potential to overlook important context, and the risk of oversimplifying complex medical issues.
- Dependence on data availability, by the potential to exclude certain groups of people who don’t have data or can’t access the technology.
- Ethical dilemmas by the potential for healthcare providers to use data in ways that compromise patient autonomy and decision-making.
- Misuse of data by the potential for data to be used to justify discriminatory or harmful decisions.
The potential drawbacks and ethical concerns
- Privacy and security concerns by the risk of breaches and unauthorized access to sensitive patient information.
- Bias and discrimination by the potential for algorithms to perpetuate and amplify existing biases, especially if the data used to train them is biased.
- Lack of transparency and accountability by the black box nature of some AI/ML models, making it difficult to understand how decisions are being made.
- Fairness concerns by the potential to reinforce existing disparities in health outcomes among different population groups.
- Lack of transparency and accountability by the black box nature of some AI/ML models, making it difficult to understand how decisions are being made.
- Overreliance on data by the potential to overlook important context, and the risk of oversimplifying complex medical issues.
- Dependence on data availability, by the potential to exclude certain groups of people who don’t have data or can’t access the technology.
- Ethical dilemmas by the potential for healthcare providers to use data in ways that compromise patient autonomy and decision-making.
- Misuse of data by the potential for data to be used to justify discriminatory or harmful decisions.
Current regulations and guidelines
- HIPAA (Health Insurance Portability and Accountability Act) is a federal regulation that sets standards for protecting sensitive patient information, including electronic protected health information (ePHI).
- FDA (Food and Drug Administration) has issued guidance on the use of real-world data in regulatory decision-making and the use of AI/ML in medical devices.
- NIST (National Institute of Standards and Technology) has issued guidelines for the development, deployment, and maintenance of trustworthy AI systems.
- HITECH (Health Information Technology for Economic and Clinical Health) Act provides funding for the implementation and use of electronic health records (EHRs) in the United States.
- GDPR (General Data Protection Regulation) is a regulation in the European Union that governs the collection, processing, and storage of personal data.
- CCPA (California Consumer Privacy Act) is a regulation in California that requires companies to disclose what personal information they collect, how they use it, and whether they disclose it to third parties.
- Industry-specific guidelines, such as the American Medical Association’s guidelines for the use of big data in medical research, and the American College of Radiology’s guidance on the use of AI in medical imaging.
Best practices for using big data and analytics in healthcare
- Data governance and management by establishing policies and procedures for data collection, storage, and use, and ensuring that data is accurate, complete, and up-to-date.
- Patient-centric approach by involving patients in the design and development of big data and analytics projects, and ensuring that their privacy and autonomy are respected.
- Transparency and communication by being open and transparent about how data is collected, used, and shared, and providing easy-to-understand explanations of how decisions are made using data.
- Fairness and explainability by ensuring that data and AI/ML models are not biased and are explainable, so that patients and healthcare providers can understand the basis for decisions.
- Ethical considerations by ensuring that data and AI/ML models are used in ways that respect patient autonomy and dignity, and that do not perpetuate existing disparities in health outcomes.
- Collaboration and partnerships by working with other healthcare organizations, researchers, and patient groups to share data and expertise, and to develop best practices for using big data and analytics in healthcare.
- Regular evaluation and monitoring by regularly monitoring the performance of AI/ML models, and making adjustments as needed to ensure that they continue to perform well and that any issues are addressed.